As a society, we are charting a course in the realm of Artificial Intelligence (AI), including in higher education. Each institution determines its own rules regarding the use of AI for submitted work or assessments. The regulations vary widely, and some institutions lack adequate policies altogether. In fact, some are still deciding whether to allow AI at all. To create sound policies, it is crucial to clarify what AI entails in education, which is what this blog aims to do.
First: what do we mean when we say AI? AI refers to applications capable of performing tasks that would typically require (human) intelligence. While the exact definition of intelligence is debatable, it is important to understand that these applications use models trained on vast datasets. Examples include systems that recognize objects in photos or videos, predict crowd density in a region, estimate epidemic risks, or provide insights into student success.
The rise of tools like ChatGPT has popularized a specific type of AI known as Generative AI (GenAI). This AI can independently generate content such as text, images, videos, programming code, Excel formulas, PowerPoint presentations, and more. The process is simple: you type an assignment (a "prompt") into the application, and it delivers a result—either on-screen or as a downloadable file.
This blog focuses specifically on the use of GenAI in higher education. The possibilities seem endless. Students can draft treatment protocols, summarize or translate large volumes of text, automatically convert data into suitable graphs or videos (with subtitles or voiceovers), and even compile theses. Likewise, teachers can use AI to assist with lesson planning, create practice questions, or even generate entire exams, including example answers and grading rubrics.
GenAI in higher education
But is this desirable? A critical question is: What does a student actually learn when AI generates materials and products? Let’s consider Miller’s Pyramid model, which describes student development toward professional competence. In the first two stages, students acquire knowledge (Know) and apply it (Apply). In the subsequent stages (Show and Do), they ultimately demonstrate their abilities in authentic professional situations. Applying knowledge involves a range of cognitive skills, such as explaining and justifying choices, validating whether the outcomes align with expectations, and so on. This is the foundation for assessing whether a client, patient, or customer receives a solution that fits their needs and context.
Students can progress through these stages—and genuinely learn—while using GenAI. Why? Because a basic assignment produces a basic result from GenAI. Therefore, crafting a highly specific assignment (a "prompt") is critical. Let’s take a detour to ChatGPT. This is an application that allows you to set up a conversation. The "Chat" part enables you to input an assignment, including additional material if needed—such as a PDF document—and receive a result. GPT stands for Generative Pre-trained Transformer. Pre-training in this context means searching for common patterns in texts. The technology used for this is called the Transformer, which scans billions of text documents. Once this pre-training process is complete, a so-called Large Language Model (LLM) is available, which can generate new text. For example, imagine you give ChatGPT the following assignment:
"Write a blog about the impact of the EU AI Act on education"
The result will then be a text in a neutral style with a few general points from the EU AI Act and a vague statement such as: "For educational institutions, the AI Act means they need to be aware of the AI technologies they implement and ensure these comply with the new regulations." Indeed, a basic assignment, or prompt, produces a basic result! That’s why you need to prime ChatGPT, meaning you provide it with contextual information. Here are some general rules for this:
- Clearly define the task.
- Specify the role in which the AI should operate.
- Outline the steps the AI should follow to complete the task.
- Provide context (e.g., relevant details, constraints).
- Specify the goal and the target audience for the result.
- Define the format for the output.
For example, a more specific prompt would be:
"Write an inspiring and action-oriented blog on the impact of the EU AI Act on higher education. You are a program manager for an ICT program at a university. Begin with a short summary of the AI Act, highlighting its key consequences. Discuss how these consequences affect higher education. Conclude with three actionable steps higher education can take to prepare for the AI Act, focusing on student assignments and assessments. The blog should be aimed at teachers, management, and leadership to inspire concrete policy development. Use clear sections, bullet points, and no technical jargon. Limit the blog to 1,000 words."
The result would be much more concrete and actionable.
The process of making a prompt as specific as possible is called prompt engineering. This brings us back to Miller’s Pyramid model. To create a specific prompt for a specific problem, basic knowledge is required and must be applied. All the elements of a specific prompt demand various analyses: the problem, tasks and responsibilities, target audiences, etc., must be understood or clarified. This forms the foundation for designing the prompt. Finally, it is crucial to interpret the result of that prompt. A Generative AI system always provides a result, but its quality and potential pitfalls must be critically evaluated and validated.
Timeliness
Could ChatGPT have written the section of this blog about the EU AI Act and higher education? Not really. One of the pitfalls of Generative AI is timeliness. A GenAI system is trained on material up to a certain date. The above prompt was used with ChatGPT based on GPT-4, which was trained on data up to October 2023. So, although the result of that prompt was more concrete and specific than the basic prompt, it does not align with the current state of affairs. After all, the EU AI Act (now officially the European Regulation on Artificial Intelligence) has only been in effect since August 1, 2024. I assume that no universities of applied sciences were actively addressing the consequences of the EU AI Act before October 2023. Therefore, ChatGPT would not have identified specific patterns during its training regarding a direct relationship between the EU AI Act and higher education. This means that the result of the prompt must be critically assessed.
What are other pitfalls? While it is becoming increasingly clear where GenAI creators source their data (for example, the billions of documents used for ChatGPT), it is also evident that certain continents or countries are underrepresented. The data comes from a variety of sources, such as (scientific) books and articles, websites, databases, forums, and social media. This has implications for the accuracy, reliability, and validity of the data. Moreover, consider all the data generated by GenAI itself based on user prompts. This data flows back into the pre-training process and eventually becomes part of the large language model (LLM), including text generated through hallucinations. GenAI tends to produce results, even when there is only a weak or no connection to the input. Additionally, while a GenAI can now provide references for its outputs, these are often general and non-specific, with the AI frequently stating that the information is based on general knowledge. This brings us to an important connection with assessments.
This raises several issues: Did the student independently create the work submitted for assessment? Or, more subtly, if the student claims AI was used to produce the result, but it turns out that work from others has been cited without proper attribution, is that considered fraud? In the first scenario, there are several considerations. The simplest approach might be to ban the use of AI altogether. But does that align with the direction in which the professional field is evolving? No. AI is now an integral part of modern society, and the workforce is well aware of this. Therefore, it is essential to ensure that future employees can use AI responsibly. For education, this may require a (re)consideration of final qualifications and learning outcomes. How do we ensure that these align with a future where AI is used as a tool—not as a replacement for human skills and decision-making? Given this, how should assessments be designed? Here too, a balance must be struck: When is it appropriate to allow AI use, and when is it not? A policy-based approach could require students to disclose whether AI was used, for what purpose, and which AI tool was employed. There are various suitable assessment formats to evaluate essential knowledge and skills. For instance, portfolio assessments and reflective reports based on peer reviews are highly effective in determining whether a student has independently interpreted, reasoned, and evaluated at the required level. If AI is prohibited as a tool, traditional assessment formats remain available. For digital assessments, tests can be conducted in secure environments (with no internet access).
There is now a wealth of resources available to assist in (re)formulating appropriate final qualifications/learning outcomes and responsibly integrating AI into education. For example, the Digital Competence Framework for Educators (DigCompEdu) outlines competencies related to digitalization at various levels of proficiency, and UNESCO has published the “Guidance for Generative AI in Education and Research.” At the national level, the Dutch government—one of the first EU member states—presented its vision on generative AI in January. Additionally, various education-related organizations, such as SURF, provide frameworks, and many universities and universities of applied sciences have developed guidelines for the responsible use of AI in education.
Putting knowledge into practice
But are we there yet? To address this, let’s revisit the EU AI Act, which officially came into effect on August 1. This Act applies to both the development and use of AI. As a regulation, it translates into legislation, which will be partially enforced starting in February 2025. Peter Frans Oosterbaan, a specialist in data and AI literacy, recently highlighted Article 4 of the Act on LinkedIn: "Providers and users of AI systems shall take measures to ensure, as far as possible, an adequate level of AI literacy among their personnel and other individuals operating and using AI systems on their behalf. They must also consider the technical knowledge, experience, education, and training of these individuals, as well as the context in which the AI systems will be used, and the people or groups of people the AI systems will impact." This means that, even when AI systems are used, an adequate level of AI literacy among users is essential. I wonder how far we have progressed on this front in higher education. The frameworks and guidelines are in place—now it’s time to activate professionalization. This professionalization effort involves more than just reading about the possibilities and pitfalls of AI; it’s about actively putting knowledge into practice.
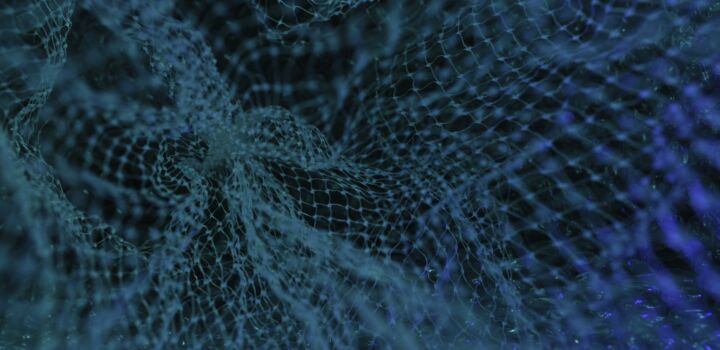
Data Science
The Data Science research group carries out applied research into the creation of data products. It focuses particularly on products that are important in a delta area such as Zeeland, for example for coastal protection, safety, tourism, food, industry, energy and logistics. The lectorate works together with entrepreneurs, governments and other research groups so that data products can be combined with knowledge from these domains.
Read more about the lectorate Data Science